🔮 E04: No10 Downing Street and Vector Databases (titles you never expect)
Shorter format today, mainly about vector databases and enabling the AI stack + some stuff on driving investment and innovation in the UK tech sector + little bit on fusion, DeFi, DAOs
This is a weekly newsletter about deep tech. Do you love deep tech? Do you love social media? Do you love sharing deep tech stuff on social media? Click the link.
I’m iterating on format; the last few have been too long (to quote a reader: “are you joking? Nobody has that much time”). Fair. This is shorter. If someone wants MORE, then head over to https://www.stateofthefuture.xyz.
Anyway, here I am, inching closer to power:
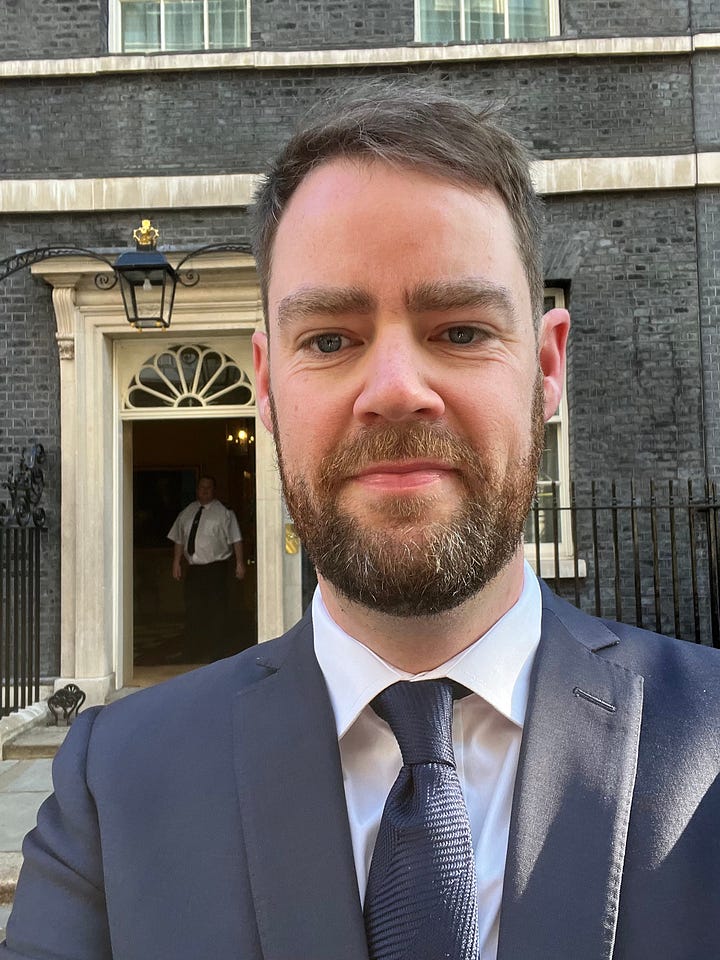
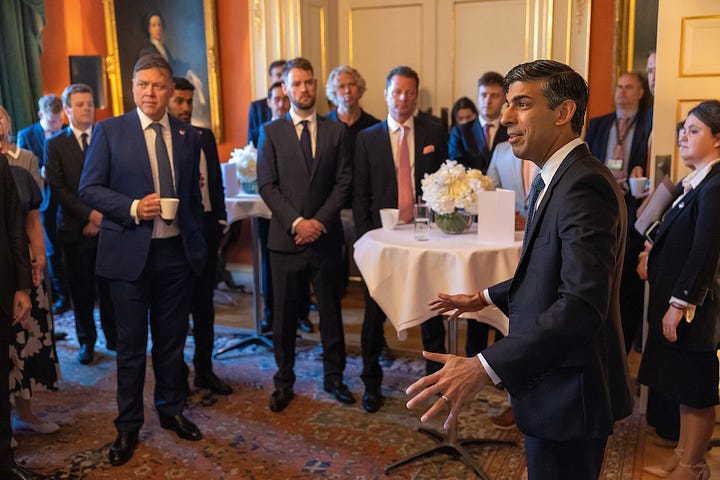
hashtag blessed to have met the Prime Minister, Rishi Sunak, at No10 Downing Street this week to discuss opportunities for driving investment and innovation in the UK technology sector. Great signalling from Rishi and gives me an opportunity to signal too. I find signalling rather than delivering to be easier. Not sure if that’s going to be true of the current Government. The good news is that everybody in the Conservative Party is focused and delivering for the British public #politics.
What are the opportunities for driving investment and innovation in the UK technology sector?
Deep Tech: Politically, I think the UK Government and any Government should care about deep tech. The Chinese figured it out a decade ago. Semiconductors. Space. ClimateTech. Materials. Mining. Robotics. Quantum. Cryptography. AI. All dual-use and where we can expect the majority of economic growth to come from in the coming decades. But the state shouldn’t pick winners, right? Sounds awfully like industrial policy. I dunno man. The Inflation Reduction Act? Lots of policy levers to pull to encourage private capital into these areas. I would think about a new class of SEIS and EIS for deep tech with bigger tax breaks. UK pension funds will be able to invest in UK startups next year; I would look to encourage more funding into deep tech somehow.
Series B Gap: I worry that pension funds will come in with all the money and neglect to take risks. Venture capital is risk capital. Or it should be. I think in the UK and Europe broadly, we have good pre-seed and seed coverage. And if startups have whatever the agreed ARR number is for B, then there is plenty of scale money. But for the really big risky things that we should find a way to fund, like new propulsion technologies for deep space exploration or new semiconductor designs, then startups still have to go to the US. Is there some way to combine the British Business Bank, British Patient Capital, and some other state-owned pension funds like the railways into a sovereign wealth fund? Could the mandate focus on deep tech from Series B? Yes, I think we could. And yes, I think we should.
Crypto? …
You simply cannot go into a Number10 and advocate for crypto. I guess a16z did, but it’s easier with $35 billion in your pocket. But, but, zero-knowledge, decentralized computing, DAOs?! Honestly, I am still long-term fascinated by the technologies like any self-respected disrupter but we need to wind it in until all the criminal activity has been washed out….
❌ Mailbag
I’m blowing up over on Linkedin, so many followers, so many likes, it won’t be long before I am a Top Voice and my immortality is secured. But remember, folks, it will never be enough. It. will. never. be. enough.
Fusion
…You might still be right in the end, but I think you may make 1 slightly incorrect assumption on fusion: that it has to achieve full commercialisation within VC time horizons. Biotech companies have often blessed VCs with returns and exits before they hit the market. I'm not saying this will happen or that biotech and fusion are the same thing. But a fusion startup showing good "pre-commercial" metrics may be able to either list or get acquired by a cash rich, prospects poor oil company, or flip into a private equity backed venture and liquidate its early backers. So far I've not made a fusion investment, but if I did, it would be in this perspective @Francesco (🇺🇦) Perticarari
…If I may protest, I see your point overall on fusion not giving commercial returns in a timeframe relevant to VC. But I see that as an issue with the definition of VC that's evolved, not fusion. Also, even within that there are possibilities of spectacular returns. If a startup were to solve the problem of how to make the "first wall" (to use your example) the valuation that could be placed upon that solution would be staggering. Even though there wouldn't yet be the rest of a nuclear reactor to put it in, that startup could have a stunning exit. @Alistair Baillie
Both have basically the same point which is great pushback. My overrated statement is specifically overrated for venture capital funds with a 10+2 structure! This statement doesn’t hold for an evergreen fund. And that the vast majority of value creation hasn’t been captured in 10+2 years. Sure, I guess you could sell on the secondary market after some value inflection point (maybe solved the first wall problem or something). My concern with that strategy is simply the depth of liquidity and the timing problem. You are basically a forced seller at a time when a small proportion(?) of the value that will be created by the company has been captured in a valuation.
So maybe I need to be clearer, the overrated, underrated and correctly rated scores are specific to 10-year closed-end venture capital funds. If I ran a family office, I would put all the money into nuclear fusion (and deep geothermal) (not investing advice). But still, what materials are we using that can get pummelled by neutrons for 20 years? (On this please do @ me. And please don’t just say, “we will figure it out”. That’s gambling, not investing.)
Bitcoin
lol. lots of people didn’t like my take. [[Bitcoin]]
DAOs
A good friend of mine [redacted] asked me: how can you think DAOs are undervalued, and DeFi is overvalued? Good question. On DeFi, my model of the world is that the app layer stuff will all be regulated and the protocol infrastructure layer stuff will not. Anyone will be able to plug into the plumbing. Banks and “trusted” financial institutions will probably plug into it too. They will probably win because of inertia and default bias, and most people want to call a number when things go wrong. And they 100% do not want to write down their private keys or figure out what a cold storage is. So will banks use Ethereum. Yep. Will DeFi be censorship-resistant? Maybe at the margins, but if you want to do anything sensible in the real world, then no. It will be regulated and censorable. So overvalued.
But DAOs. Almost all DAOs are “communities with tokens”. That’s not what I call a DAO. Bitcoin is a DAO. Ethereum is a DAO, now. DAOs have to be uncensorable. Otherwise they are DOs. The devil is in the detail. You need lots of active members/nodes/token holders with *relatively* dispersed ownership. I don’t know what the right threshold is for *relatively*, but I do know it’s not 10% to three founders, and 30% to VCs. Now all the DeFi claims they are DAOs now and issued governance tokens in an attempt to skirt around the coming/arrived regulatory clampdown. But that’s a Kansas City Shuffle, not a DAO. The power of a real DAO is that they are the only real organising structure for unstoppable, uncensorable, permissionless protocols. They are the first digital-first, global-by-design, mechanisms for allocating resources. I don’t see a pathway where they don’t become ubiquitous to digital-only trade and digital-only AI trade, too. So undervalued. (Biases: investors in samudai and molecule)
Tell me what I am overweighting, underweighting, or falsely assuming in the chat:
Now State of the Future is effectively a database of 100+ technology assessments. I’ll share a different one every week. But this week, after a few people reached out to me and asked what I thought of vector databases, I thought I would write it up.
The database for AI? Or another hackernews fan favourite?
✍️ Vector Databases
Summary
summary 1: explain it to me like I’m 15
Picture this: You have a vast collection of songs, Leave The Water Still by Sonnee, Friday Night by orbit, Escalate by Ben Bohmer and All My Life by Lil Durk, just to pick some random examples. You want to organize them according to their genre and mood. Vibing, Beast Mode, Drown out the Kids, etc. Again some random examples. Now, data management has a similar challenge with pieces of information like text, images, and sounds.
1. Embeddings: Think of embeddings as a sophisticated method of categorizing or tagging information. For instance, a happy pop song and a sad classical piece need different tags. Embeddings convert information, such as words or images, into groups of numbers in a way that similar information gets similar groups.
2. Vectors: These groups of numbers are known as vectors. It's like taking the essence of the information (e.g., the mood and genre of a song) and representing it as points on a graph. The beauty of vectors is that similar ones are close to each other on this graph.
3. Large Language Models: LLMs use embeddings to deeply understand the context and semantics of the text. These models can write, answer questions, and have conversations because they can swiftly sift through vectors that represent an enormous amount of text information.
4. Vector Databases: Picture a well-organized bulletin board where you can pin your song tags. All My Life by Lil Durk next to 30 by Nas, for example. A vector database is akin to this bulletin board (do kids know what a bulletin board is anymore? tough luck I guess). It's a storage system designed to efficiently store and retrieve vectors. When an AI needs to find similar pieces of information, the vector database allows it to quickly find the relevant vectors, much like you would quickly find songs by looking at tags pinned closely together on the bulletin board.
So, in essence, embeddings and vectors are tools to tag and plot information, LLMs use them for text understanding, and vector databases are the organized boards that keep everything easily accessible.
summary 2: explain it to me like I’m a deep tech investor
A vector database is a specialized type of database that employs mathematical operations within vector spaces to manage data. It is designed for handling high-dimensional data, a form that is ubiquitous in machine learning and AI applications. Here, a 'vector' signifies a mathematical depiction of data within a space that can span multiple dimensions. Each dimension encapsulates a distinct feature or characteristic of the data. As the dimensionality or the number of features increases, so does the complexity of managing and interpreting the data, a challenge often referred to as the "curse of dimensionality." Therefore, to efficiently store, search, and retrieve high-dimensional data, we rely on specialized tools, such as vector databases.
(i) viability: how mature is the technology? (5)
Vector databases are in the commercialization phase, with a growing market and increasing investment in the technology. The global vector database market is still small in the order of something like $1.0 billion. The rapid rise of LLMs has put vector databases in the spotlight as they make data more accessible for AI systems, potentially enhancing their reliability. R&D focuses on scaling and enabling vector databases to manage every larger models. There is also a growing trend of integrating generative AI with vector databases. An example is KX's KDB.AI, designed for cloud-native vector data management, vector embeddings, and GPT-like queries. Another is combining ETL (extract, transfer, and load) and real-time data streams in the preparation stage before vector embeddings are encoded into the database.
(ii) drivers: how powerful are adoption forces? (5)
On the supply side (the technology), advancements in vector databases are largely driven by progress in vector search algorithms. Key algorithms such as HNSW (Hierarchical Navigable Small World) and ANNOY (Approximate Nearest Neighbors Oh Yeah) have significantly improved search efficiency and accuracy. Simultaneously, GPU performance and Cloud computing improved to provide sufficient computing power to enable efficient vector databases. On the demand side (customers), increased unstructured data and semantic understanding necessitate better data management solutions and interest in vector databases. But the introduction of LLMs has increased growth rates from <10% to maybe 50% annually. In summary, vector databases like human extinction are now hot because of LLMs.
(iii) novelty: how much better relative to alternatives? (3)
Vector databases compete with other databases in handling unstructured and high-dimensional data. Vector databases are better than NoSQL databases at handling high-dimensional data, but the cost of using a new database may not be worth it for many customers. On the performance side, for example, graph databases can model complex relationships in high-dimensional data but lack native support for vector operations. Distributed data processing tools like Hadoop can handle large volumes of high-dimensional data, although they aren't databases in the traditional sense. Time-series databases like InfluxDB could handle high-dimensional data with a temporal component. The performance advantages of vector databases might decrease if NoSQL databases add more features to compress data like lemmatization, stemming, TF-IDF (Term Frequency-Inverse Document Frequency), and fuzzy queries, all of which will make information retrieval and analysis more efficient without the need for a new database.
(iv) diffusion: how easily will it be adopted? (5)
The main restraints to adoption are fairly common to new software infrastructure and all relatively minor. Most of the problems are around lack of knowledge, lack of standardisation, scalability, cost and interoperability. All of these issues are likely to be solved quickly (<12 months) as winners emerge quickly serving the most used LLMs and become de facto standards. Open-source variants like pgvector are already spreading quickly enabling low cost and speedy experimentation. The one issue may well be cost as hardware resources become more scarce and costly. The so-called GPU crunch. Or rather Nvidia tax. Or rather A100 crunch. But that won’t quell overall demand rather, delay it slightly. Hard to make any strong case that adoption won’t be really fast for the target LLM use case.
(v) impact: how much value is created? (4)
Medium certainty: The impact of vector databases depends on how much better a vector database is than a NoSQL database with added "compression" features for data storage and retrieval. It does seem most likely that for LLMs, a vector-type functionality is a prerequisite for the performance. I'm minded by some research I did last year: "roughly 50% of organisations are still using a single relational database?! 30% using a relational database and MongoDB, and 20% using a relational + MongoDB + some best-in-class NoSQL database." So short-term, vector databases are going to see adoption for what is a small, albeit fast growing niche of LLMs. The high impact scenario is that vector databases enable LLMs, and LLMs are one of/the most impactful technologies of the decade/ever. They become a key part of an emerging "AI stack" with other parts like distributed computing systems like Kubernetes and Apache Spark; frameworks like TensorFlow, PyTorch, and JAX; and serving systems like TensorFlow Serving or Triton Inference Server. A low-impact scenario would see that LLMs are overhyped, the market will be a niche and multi-model databases with all "vector-like" features are good enough to handle high dimensional data for most of the market.
(vi) timing: when will the market be large enough or growing fast enough for risk capital? (Now: 2020-2025)
High Certainty: The vector databases market is already attracting significant venture investment. The global vector database market size was worth something like $1.0 billion in 2021, but that was before LLM demand. I would expect a $10 billion market by 2026. Certainly large enough to support venture capital. As of April 2023, vector database startups had raised over $350 million to build generative AI infrastructure. It wouldn’t surprise me to see that number grow to $750m as VCs realise the opportunity to be the database for AI. For example, in April, Pinecone raised $100 million at a $750 million valuation, and Weaviate raised $50 million at a $200 million valuation. While Zilliz has amassed a total investment of $113 million, and Milvus and Chroma have also raised significant funding. The race is on.
2030 Prediction
Multi-model databases add vector-like features as vector databases are a niche albeit valuable segment like graph databases.
Open Questions
The database market tends towards general-purpose databases because database migration is hard and no one wants to do it. It is *materially* easier to add new features from your existing database than buy and integrate an entirely new one. Inertia is very powerful. Is this time different because LLMs are so useful? My prediction tends towards a multi-model outcome.
Does inertia even matter? Vector databases are going after startups and younger companies for whom database migration is less of a challenge than a Fortune 500 company that first bought IBMD2 in the 90s. Is that market large enough anyway?
Even if vector databases are not widely adopted, will they have a massively outsized impact because they enable LLMs, which will impact the economy dramatically? How should we consider “impact” in terms of allowing a general-purpose technology but remaining relatively niche? Does a score of 4 capture that?
Update:
I read this just a moment ago:
“88% believe a retrieval mechanism, such as a vector database, would remain a key part of their stack. Retrieving relevant context for a model to reason about helps increase the quality of results, reduce “hallucinations” (inaccuracies), and solve data freshness issues. Some use purpose-built vector databases (Pinecone, Weaviate, Chroma, Qdrant, Milvus, and many more), while others use pgvector or AWS offerings.” Source: Sequoia
Still too early to help answer the open questions, but I would read from this that a new “AI” or LLM stack will need a vector database. The question is still: will the AI stack be niche? Or will it replace/merge with the broader web/software stack?
Sources
Morris Clay’s brain,
Vector Databases, https://unzip.dev/0x014-vector-databases/
The New Language Model Stack, https://www.sequoiacap.com/article/llm-stack-perspective/
What is a Vector Database?, https://www.pinecone.io/learn/vector-database/
The Rise Of Vector Databases, https://www.forbes.com/sites/adrianbridgwater/2023/05/19/the-rise-of-vector-databases/
8 Best Vector Databases to Unleash the True Potential of AI, https://geekflare.com/best-vector-databases/
A Gentle Introduction to Vector Databases https://frankzliu.com/blog/a-gentle-introduction-to-vector-databases
r/vectordatabase, https://www.reddit.com/r/vectordatabase
Vector databases, https://db-engines.com/en/blog_post/104
Trilemma Trade-Offs: A New CAP Theorem For Vector Databases Has Emerged, https://www.forbes.com/sites/forbestechcouncil/2023/06/02/trilemma-trade-offs-a-new-cap-theorem-for-vector-databases-has-emerged/
Bye.